NVIDIA Unveils NeMo Retriever Microservices to Enhance AI Accuracy and Throughput
The post NVIDIA Unveils NeMo Retriever Microservices to Enhance AI Accuracy and Throughput appeared on BitcoinEthereumNews.com. Lawrence Jengar Jul 24, 2024 01:25 NVIDIA introduces NeMo Retriever NIM microservices, improving AI accuracy and throughput, integrated with platforms like Cohesity and NetApp. NVIDIA has announced the launch of its new NeMo Retriever NIM (NeMo Inference Microservices) designed to significantly enhance the accuracy and throughput of Large Language Models (LLMs) in AI applications. These microservices aim to help developers access and utilize proprietary data more efficiently, thereby generating more accurate and relevant responses for AI-driven tasks, according to the NVIDIA Blog. Boosting AI Accuracy with NeMo Retriever The NeMo Retriever NIM microservices are production-ready and designed for retrieval-augmented generation (RAG). This new suite of tools allows enterprises to scale AI workflows with minimal intervention, ensuring high accuracy in various applications. The microservices integrate seamlessly with platforms such as Cohesity, DataStax, NetApp, and Snowflake. These microservices are particularly beneficial for developers working on AI agents, customer service chatbots, security vulnerability analysis, and extracting insights from complex supply chain data. By enabling high-performance, enterprise-grade inferencing, NeMo Retriever NIM microservices can supercharge AI applications with enhanced data accuracy and throughput. Embedding and Reranking Models NeMo Retriever NIM microservices consist of two main model types: embedding and reranking. Embedding models transform diverse data into numerical vectors, capturing their meaning and nuances, while reranking models score data based on its relevance to a given query. By combining these two models, developers can ensure the most accurate and relevant results for their AI applications. The embedding models, such as NV-EmbedQA-E5-v5 and NV-EmbedQA-Mistral7B-v2, are optimized for text question-answering retrieval and multilingual embedding, respectively. The reranking models, including NV-RerankQA-Mistral4B-v3, provide high-accuracy text reranking capabilities. These models are now generally available and accessible through the NVIDIA API catalog. Top Use Cases NeMo Retriever NIM microservices offer a wide range of applications, from building intelligent chatbots and analyzing security…
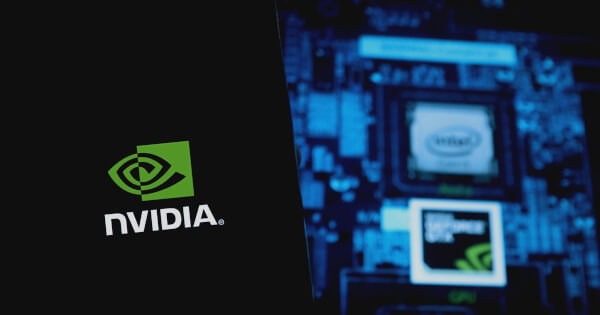
The post NVIDIA Unveils NeMo Retriever Microservices to Enhance AI Accuracy and Throughput appeared on BitcoinEthereumNews.com.
Lawrence Jengar Jul 24, 2024 01:25 NVIDIA introduces NeMo Retriever NIM microservices, improving AI accuracy and throughput, integrated with platforms like Cohesity and NetApp. NVIDIA has announced the launch of its new NeMo Retriever NIM (NeMo Inference Microservices) designed to significantly enhance the accuracy and throughput of Large Language Models (LLMs) in AI applications. These microservices aim to help developers access and utilize proprietary data more efficiently, thereby generating more accurate and relevant responses for AI-driven tasks, according to the NVIDIA Blog. Boosting AI Accuracy with NeMo Retriever The NeMo Retriever NIM microservices are production-ready and designed for retrieval-augmented generation (RAG). This new suite of tools allows enterprises to scale AI workflows with minimal intervention, ensuring high accuracy in various applications. The microservices integrate seamlessly with platforms such as Cohesity, DataStax, NetApp, and Snowflake. These microservices are particularly beneficial for developers working on AI agents, customer service chatbots, security vulnerability analysis, and extracting insights from complex supply chain data. By enabling high-performance, enterprise-grade inferencing, NeMo Retriever NIM microservices can supercharge AI applications with enhanced data accuracy and throughput. Embedding and Reranking Models NeMo Retriever NIM microservices consist of two main model types: embedding and reranking. Embedding models transform diverse data into numerical vectors, capturing their meaning and nuances, while reranking models score data based on its relevance to a given query. By combining these two models, developers can ensure the most accurate and relevant results for their AI applications. The embedding models, such as NV-EmbedQA-E5-v5 and NV-EmbedQA-Mistral7B-v2, are optimized for text question-answering retrieval and multilingual embedding, respectively. The reranking models, including NV-RerankQA-Mistral4B-v3, provide high-accuracy text reranking capabilities. These models are now generally available and accessible through the NVIDIA API catalog. Top Use Cases NeMo Retriever NIM microservices offer a wide range of applications, from building intelligent chatbots and analyzing security…
What's Your Reaction?
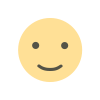
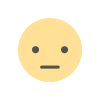




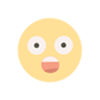