Deploying Trillion Parameter AI Models: NVIDIA’s Solutions and Strategies
The post Deploying Trillion Parameter AI Models: NVIDIA’s Solutions and Strategies appeared on BitcoinEthereumNews.com. Artificial Intelligence (AI) is revolutionizing numerous industries by addressing significant challenges such as precision drug discovery and autonomous vehicle development. According to the NVIDIA Technical Blog, the deployment of large language models (LLMs) with trillions of parameters is a pivotal aspect of this transformation. Challenges in LLM Deployment LLMs generate tokens mapped to natural language, which are then sent back to the user. Increasing token throughput can enhance return on investment (ROI) by serving more users, though this may reduce user interactivity. Striking the right balance between these factors is increasingly complex with evolving LLMs. For instance, the GPT MoE 1.8T parameter model has subnetworks that independently perform computations. The deployment considerations for such models include batching, parallelization, and chunking, all of which affect inference performance. Balancing Throughput and User Interactivity Enterprises aim to maximize ROI by increasing the number of user requests served without additional infrastructure costs. This involves batching user requests to maximize GPU resource utilization. However, user experience, measured by tokens per second per user, demands smaller batches to allocate more GPU resources per request, which can lead to underutilization of GPU resources. The trade-off between maximizing GPU throughput and ensuring high user interactivity is a significant challenge in deploying LLMs in production environments. Parallelism Techniques Deploying trillion-parameter models requires various parallelism techniques: Data Parallelism: Multiple copies of the model are hosted on different GPUs, independently processing user requests. Tensor Parallelism: Each model layer is split across multiple GPUs, with user requests shared among them. Pipeline Parallelism: Groups of model layers are distributed across different GPUs, processing requests sequentially. Expert Parallelism: Requests are routed to distinct experts in transformer blocks, reducing parameter interactions. Combining these parallelism methods can significantly improve performance. For example, using tensor, expert, and pipeline parallelism together can deliver substantial GPU throughput without sacrificing…
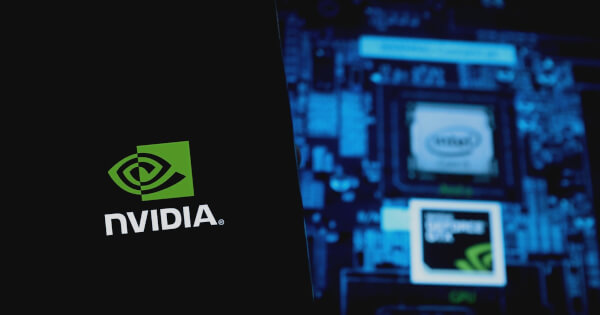
The post Deploying Trillion Parameter AI Models: NVIDIA’s Solutions and Strategies appeared on BitcoinEthereumNews.com.
Artificial Intelligence (AI) is revolutionizing numerous industries by addressing significant challenges such as precision drug discovery and autonomous vehicle development. According to the NVIDIA Technical Blog, the deployment of large language models (LLMs) with trillions of parameters is a pivotal aspect of this transformation. Challenges in LLM Deployment LLMs generate tokens mapped to natural language, which are then sent back to the user. Increasing token throughput can enhance return on investment (ROI) by serving more users, though this may reduce user interactivity. Striking the right balance between these factors is increasingly complex with evolving LLMs. For instance, the GPT MoE 1.8T parameter model has subnetworks that independently perform computations. The deployment considerations for such models include batching, parallelization, and chunking, all of which affect inference performance. Balancing Throughput and User Interactivity Enterprises aim to maximize ROI by increasing the number of user requests served without additional infrastructure costs. This involves batching user requests to maximize GPU resource utilization. However, user experience, measured by tokens per second per user, demands smaller batches to allocate more GPU resources per request, which can lead to underutilization of GPU resources. The trade-off between maximizing GPU throughput and ensuring high user interactivity is a significant challenge in deploying LLMs in production environments. Parallelism Techniques Deploying trillion-parameter models requires various parallelism techniques: Data Parallelism: Multiple copies of the model are hosted on different GPUs, independently processing user requests. Tensor Parallelism: Each model layer is split across multiple GPUs, with user requests shared among them. Pipeline Parallelism: Groups of model layers are distributed across different GPUs, processing requests sequentially. Expert Parallelism: Requests are routed to distinct experts in transformer blocks, reducing parameter interactions. Combining these parallelism methods can significantly improve performance. For example, using tensor, expert, and pipeline parallelism together can deliver substantial GPU throughput without sacrificing…
What's Your Reaction?
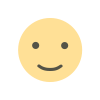
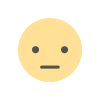




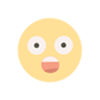